Machine learning for discovery of hidden material properties
Elasticity Imaging Using Physics-Informed Neural Networks: Spatial Discovery of Elastic Modulus and Poisson's Ratio
Summary: Elasticity imaging is a technique that discovers the spatial distribution of mechanical properties of tis- sue using deformation and force measurements under various loading conditions. Given the complexity of this discovery, most existing methods approximate only one material parameter while assuming ho- mogeneous distributions for the others. We employ physics-informed neural networks (PINN) in linear elasticity problems to discover the space-dependent distribution of both elastic modulus ( E ) and Pois- son’s ratio ( ν) simultaneously, using strain data, normal stress boundary conditions, and the governing physics. We validated our model on three examples. First, we experimentally loaded hydrogel samples with embedded stiff inclusions, representing tumorous tissue, and compared the approximations against ground truth determined through tensile tests. Next, using data from finite element simulation of a rect- angular domain containing a stiff circular inclusion, the PINN model accurately localized the inclusion and estimated both E and ν. We observed that in a heterogeneous domain, assuming a homogeneous ν distribution increases estimation error for stiffness as well as the area of the stiff inclusion, which could have clinical importance when determining size and stiffness of tumorous tissue. Finally, our model ac- curately captured spatial distribution of mechanical properties and the tissue interfaces on data from another computational model, simulating uniaxial loading of a rectangular hydrogel sample containing a human brain slice with distinct gray matter and white matter regions and complex geometrical features. This elasticity imaging implementation has the potential to be used in clinical imaging scenarios to re- liably discover the spatial distribution of mechanical parameters and identify material interfaces such as tumors.
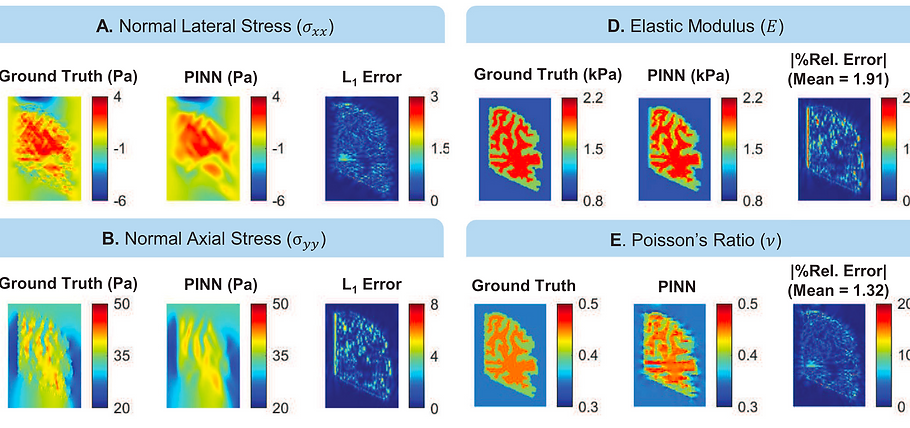
Non-invasive MRI elastography for brain's material properties
Summary: The brain’s stiffness measurements from magnetic resonance elastography (MRE) strongly depend on actuation frequencies, which makes cross-study comparisons challenging. We performed a preliminary study to acquire optimal sets of actuation frequencies to accurately obtain rheological parameters for the whole brain (WB), white matter (WM), and gray matter (GM). Six healthy volunteers aged between 26 and 72 years old went through MRE with a modified single-shot spin-echo echo planar imaging pulse sequence embedded with motion encoding gradients on a 3T scanner. Frequency-independent brain material properties and best-fit material model were determined from the frequency-dependent brain tissue response data (20 -80 Hz), by comparing four different linear viscoelastic material models (Maxwell, Kelvin-Voigt, Springpot, and Zener). During the material fitting, spatial averaging of complex shear moduli (G*) obtained under single actuation frequency was performed, and then rheological parameters were acquired. Since clinical scan time is limited, a combination of three actuation frequencies that would provide the most accurate approximation and lowest fitting error was determined for WB, WM, and GM by optimizing for the lowest Bayesian information criterion (BIC). BIC scores for the Zener and Springpot models showed these models approximate the multifrequency response of the tissue best. The best-fit frequency combinations for the reference Zener and Springpot models were identified to be 30-60-70 and 30-40-80 Hz, respectively, for the WB. Optimal sets of actuation frequencies to accurately obtain rheological parameters for WB, WM, and GM were determined from shear moduli measurements obtained via 3-dimensional direct inversion. We believe that our study is a first-step in developing a region-specific multifrequency MRE protocol for the human brain.
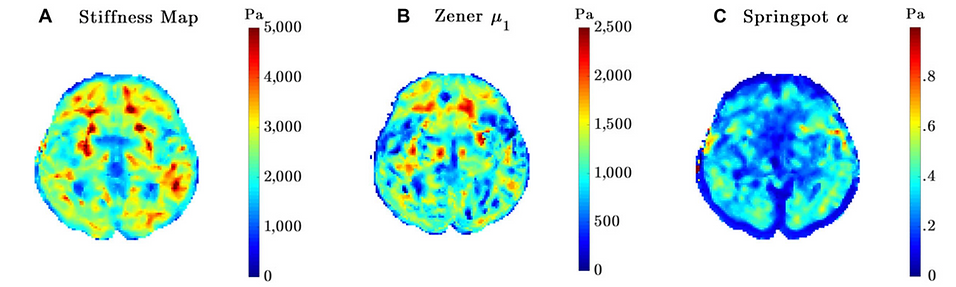
Energy-based local material characterization of canine aorta
Summary: This study aims at determining the in vitro anisotropic mechanical behavior of canine aortic tissue and its spatial variations along the axis of the vessel. Eight canine aortas were acquired to harvest circumferential and longitudinal specimens at various distal locations along the aorta’s axis. A constitutive model was developed that incorporates both phenomenological and structural elements to account for macroscopic and microstructural behavior of the tissue based on the findings of uniaxial cyclic tensile testing and histological examinations.The findings showed significant changes in mechanical behavior of the tissue as a function of axial position. The nonlinear constitutive modeling provided good fits to the experimental data and indicated regional changes in material behavior. The constitutive models can be readily used to design computational models of tissue deformation during phenomenological loading cycles. The findings of this study extend the understanding of local mechanical properties that could lead to region-specific diagnostics and treatment of arterial diseases.
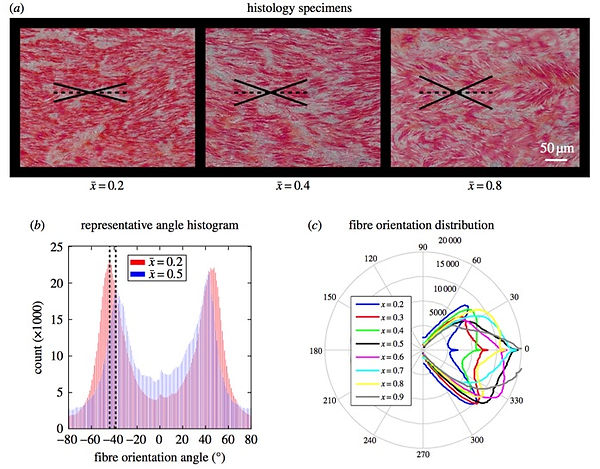